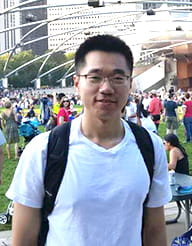
Richard Y. Chen
Department of Statistics, University of Chicago, PhD candidate
Broadly speaking, Richard’s main research efforts are statistical methodology and theory of time series analysis. Driven by a surge of low-latency data from the deluging TAQ database, his research focuses on nonparametric statistics of hidden Ito semimartingales, including noisy diffusions and Levy processes to model and analyze financial data. Previously, with Professor Per A. Mykland, he published a work investigating the impact of non-stationary microstructure noise on two-time scale realized volatility estimator (TSRV), proposing asymptotically powerful statistical tests, and found new evidence on noise non-stationarity in NYSE S&P 500 components. Recently, he worked on volatility functional inference using noisy data, motivated by their important applications in measuring economic risks and testing econometric models, e.g., factor analysis, estimating betas, generalized method of integrated moments. In particular, by combining the strengths of two strands of fundamental works, Jacod et al. (2009, 2010) and Jacod, Rosenbaum (2013), a new inferential framework has been developed with time-domain noise dilution, jump truncation, and correction for high-order nonlinearity biases, enables applications harvesting data at a higher frequency than previous literature allows.
Around the time of this fellowship award, Richard Y. Chen works on an adaptive enhancement of volatility functionals for possible efficiency gain; Fourier-Malliavin type of methods combined with Ito calculus for measuring empirical properties of stochastic volatility, including spot volatility in time domain and possible frequency-domain perspectives.
Richard is a 2019 Stevanovich Fellowship recipient.